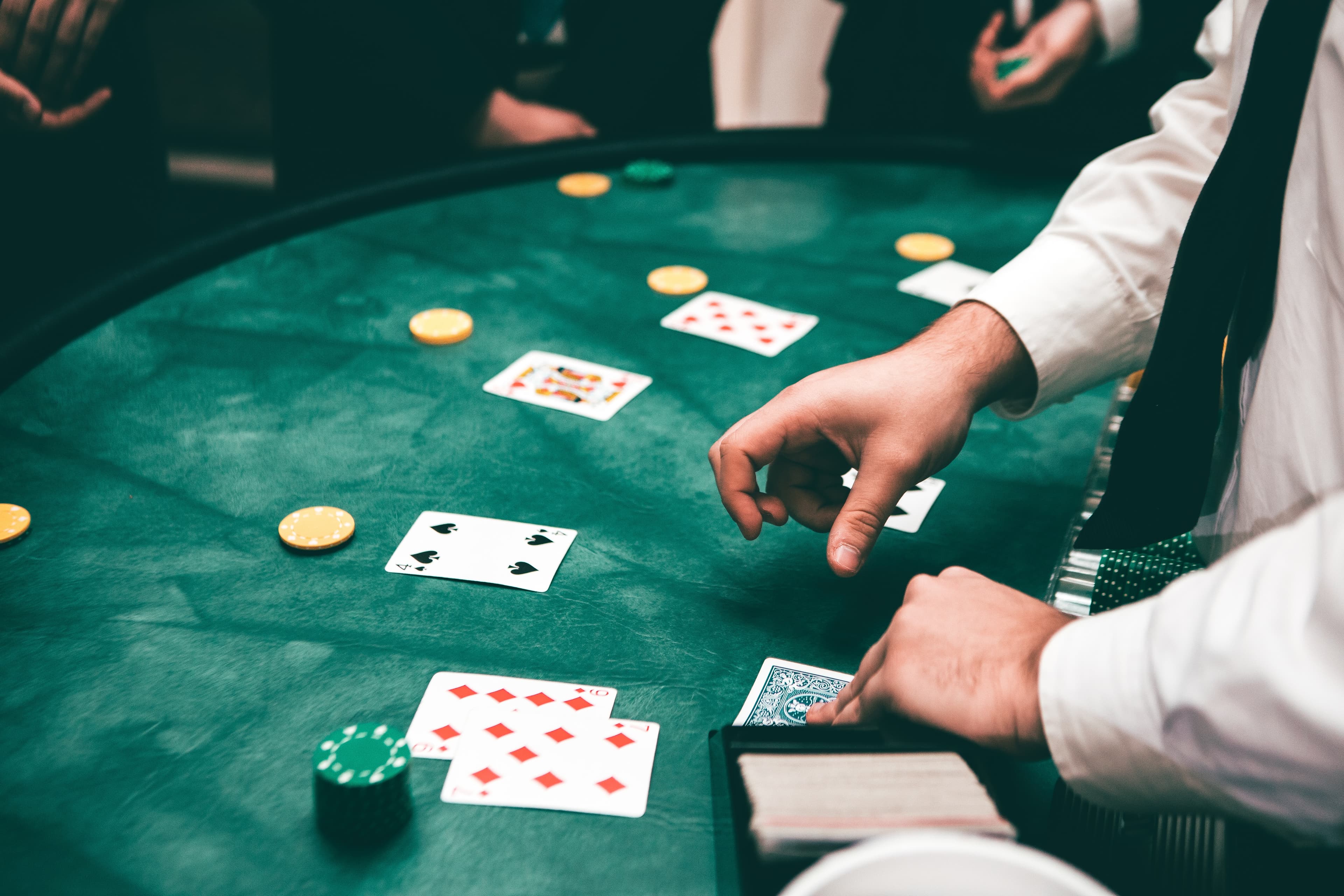
23.5.2023
Blackjack Surveillance for detecting Card Counters
Szymon Fonau, CTO @ OctoShrew
Introduction
The world of blackjack has long been a battleground where players strive to outwit the casino, and casinos, in turn, employ various strategies to maintain their advantage. In this case study, we delve into the development of a groundbreaking blackjack surveillance algorithm that revolutionizes the way casinos detect card counters and predict the house edge for each player. This algorithm combines cutting-edge technologies such as machine learning, computer vision, and facial recognition to provide an unprecedented level of insight and control over the game.
Objective
The primary objective of this algorithm is to identify card counters and accurately estimate the house edge for individual players based on their gameplay patterns. Card counting, a technique used to gain an edge over the casino, has long been a challenge for casinos to detect. By utilizing sophisticated machine learning techniques, the algorithm can identify players who exhibit a negative expectation value, signaling potential card counting behavior.
Features
In addition to card counting detection, the algorithm incorporates computer vision capabilities to track the movement of chips on the blackjack table. This allows for accurate monitoring of bet amounts and player actions, enabling the system to generate predictions on the house edge for each player. Moreover, the algorithm employs facial recognition technology, ensuring that players can be identified even if they switch tables, further enhancing surveillance capabilities.
Development
The development process of this blackjack surveillance algorithm involved extensive data collection, preprocessing, and feature extraction. Gameplay data from multiple tables was gathered, and computer vision algorithms were employed to capture images and videos of players. Subsequently, machine learning models were developed to predict the house edge for each player and detect card counting patterns. The algorithm was rigorously tested and refined based on feedback from surveillance staff to ensure its accuracy and reliability.
Outcome
The implementation of this algorithm brings numerous benefits to both the casino and the players. Casinos can enhance their surveillance efficiency by accurately identifying card counters and suspicious players, reducing losses caused by skilled gamblers. The ability to predict the house edge for individual players allows casinos to optimize table limits and allocate resources effectively. Furthermore, players benefit from a fair and secure gaming environment, as the algorithm prevents unfair advantages through card counting and enables personalized services based on facial recognition.
Conclusion
In conclusion, the development of this blackjack surveillance algorithm marks a significant advancement in casino surveillance technology. By leveraging machine learning, computer vision, and facial recognition, the algorithm empowers casinos with a comprehensive understanding of player behavior and enables them to maintain their edge. The subsequent sections of this case study will delve into the development process, algorithm functionality, and the potential impact of this innovative solution on the casino industry and player dynamics.
Related blogs
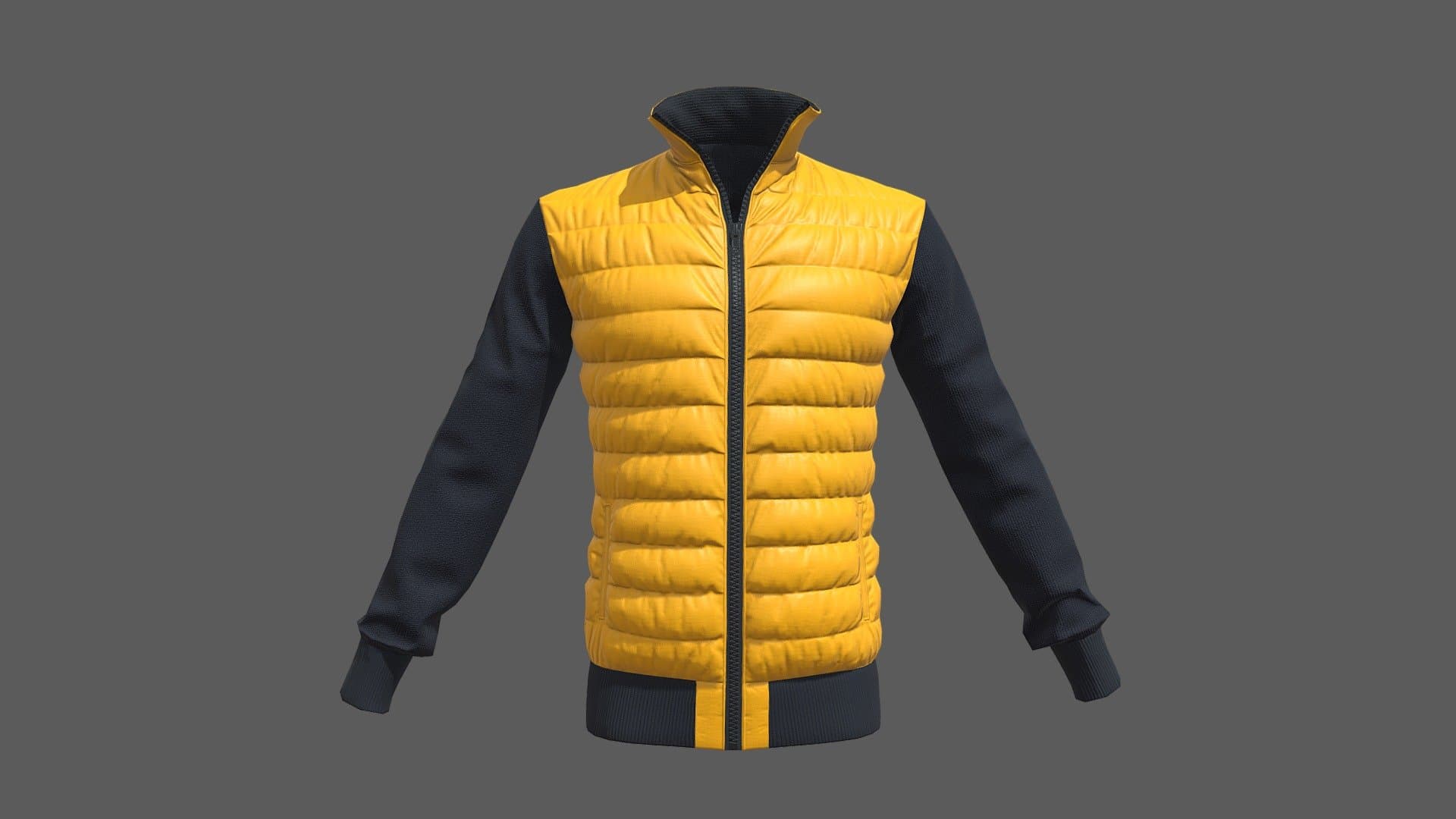
23.5.2023
3D Clothing Reconstruction for Online Marketplaces
Mayank Laddha, Machine Learning Engineer @ OctoShrew
case-study, shopping, deep-learning, computer-vision, machine-learning
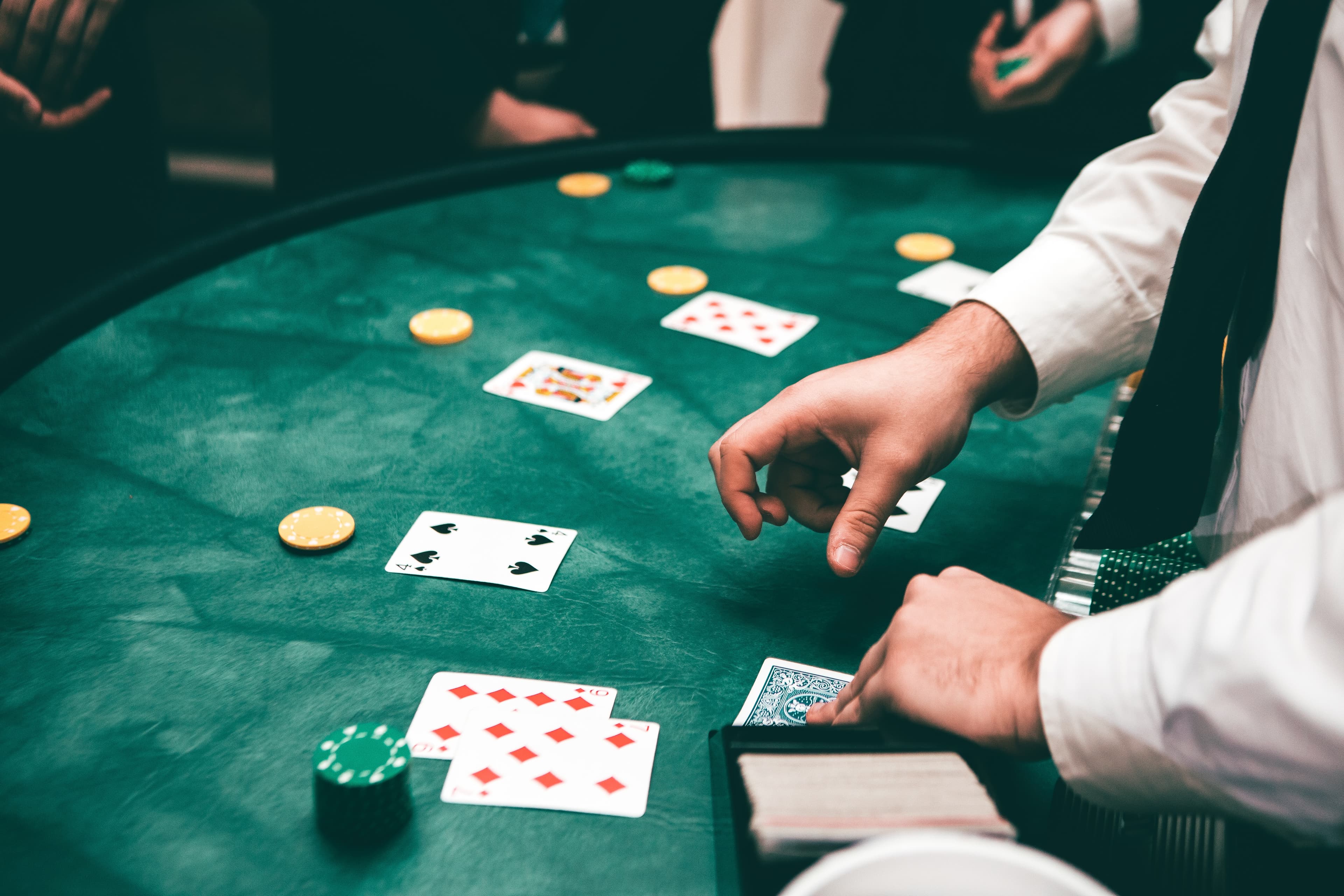
23.5.2023
Blackjack Surveillance for detecting Card Counters
Szymon Fonau, CTO @ OctoShrew
case-study, data-science, computer-vision
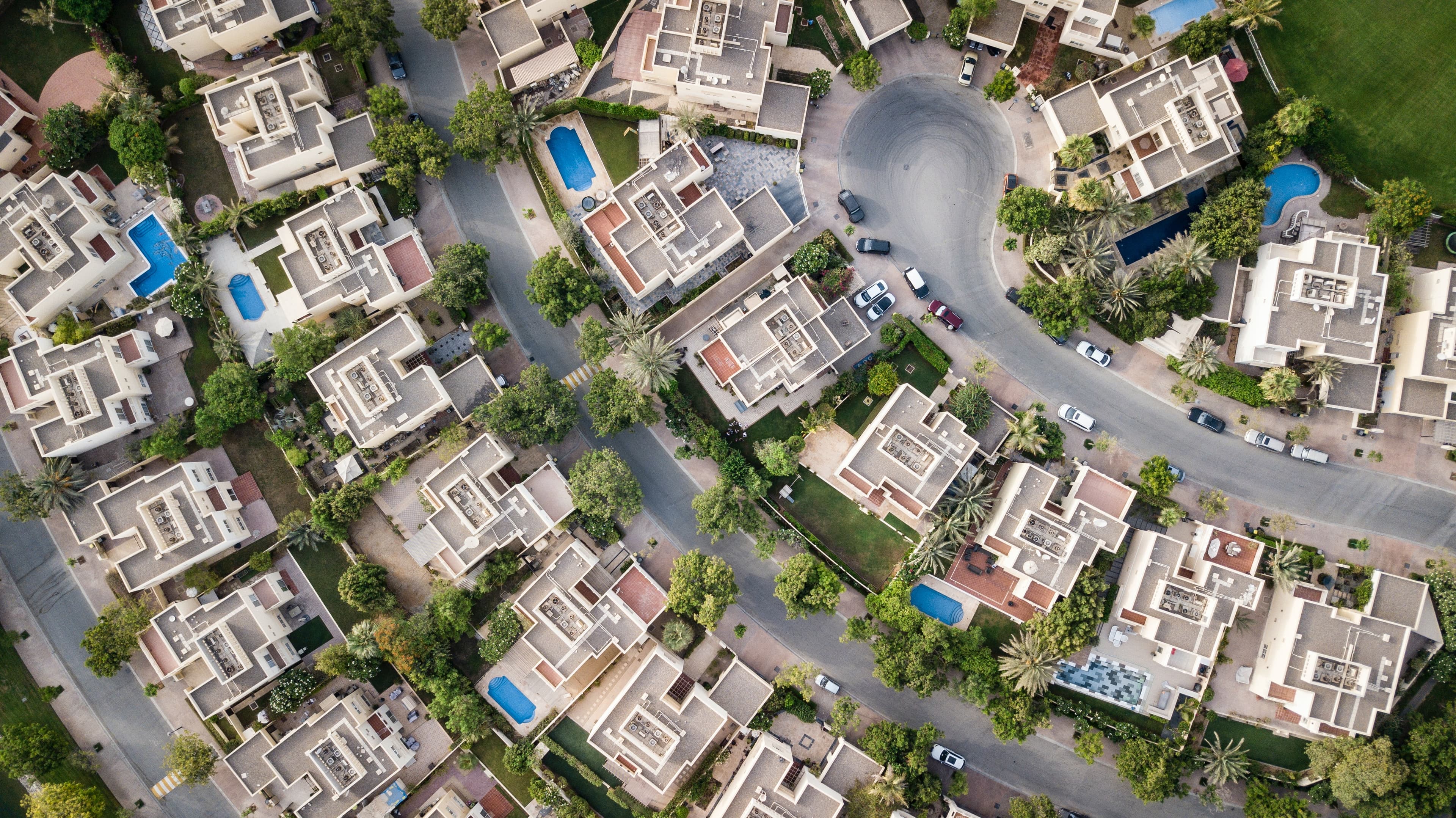
23.5.2023
Boosting Conversions with Automated House Value Estimation
Szymon Fonau, CTO @ OctoShrew
case-study, data-science, marketing, machine-learning